By Guy D’Hauwers, atNorth
The original definition of artificial intelligence was the ability of computers to perform tasks that people usually did. However, as the technology continues to develop it is apparent that there are many situations whereby computers can collate up-to-the minute data to provide analysis that no human could feasibly do. This isn’t to say that computers can, or will, replace human jobs, but rather that they provide a better service that transforms the way in which tasks are carried out across business today.
Many companies are adjusting their business models to secure stakeholders’ trust and safeguard long-term profitability and this means that in order to stay competitive, businesses are increasingly leaning on AI to automate and optimise key processes that can increase productivity and operational efficiencies. The technology is already proving invaluable in heavily data driven industries such as research and development or banking. The power of AI now allows engineers to simulate a new vehicle in a matter of hours, or review MRI scans in a fraction of the time of a doctor – just two examples of a myriad of applications that AI can support. The results are amazing, but what is the cost?
Digitalization has had a massive impact on our climate, yielding an ever-increasing demand for electricity and rising carbon emissions because of its acceleration. To train just one computer to behave in a humanlike way via machine learning (ML) on a GPT-3 large language model can use upwards of 12mW of data and cost up to $3 million dollars, not to mention the huge amount of energy needed to power this process. And if at any time the requirements change, the process of training the computer must be repeated from scratch.
With the increase in demand for these high-density workloads, businesses have had to invest heavily in computer hardware such as graphics processing units (GPUs), which are necessary for advanced calculations for AI, natural language processing (NLP), scientific simulations and risk analysis amongst, other things.
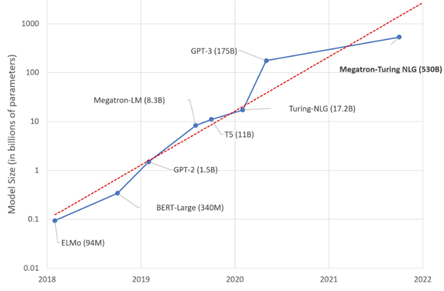
Training 1 x GPT-3 costs $3M on public cloud: • Estimated 1000 x A100 * 4.42$/h * 1 month • (AWS p4d.24xlarge, @ 35.39655 $/h) • Consumes an extreme amount of energy – 1200 megawatt hours (Patterson et. al. 2021)
Most IT departments cannot support this significant infrastructure internally. For businesses to build their own GPUs to power AI activity could take 18 months – considerably delaying any product to market – and, whilst they can be hosted in the public cloud, most companies would not deem this secure enough. Yet access to physical data centres that have the capability to handle high performance computing (HPC) may not be possible – especially if instant global data processing is required.
This is because most legacy data centres built for general purpose computing have become outdated, and are not capable of accommodating the GPU servers, density and storage capacity to manage these workloads in an efficient way. Additionally, the amount of energy required to upgrade these legacy data centres to HPC standards is vast. If every data centre upgraded to adhere to HPC specifications, the world would experience a serious energy crisis.
The nature of these applications, in addition to rising costs associated with using public cloud services and increased pressure on sustainability, has tasked many organisations with the challenge of finding new alternatives to ensure continuity with high-performance applications in a cost-effective and energy efficient way. As businesses strive to remain competitive and the demand for this kind of technology increases, there must be a way of preventing the financial, technological and ecological fallout that seems likely to occur.
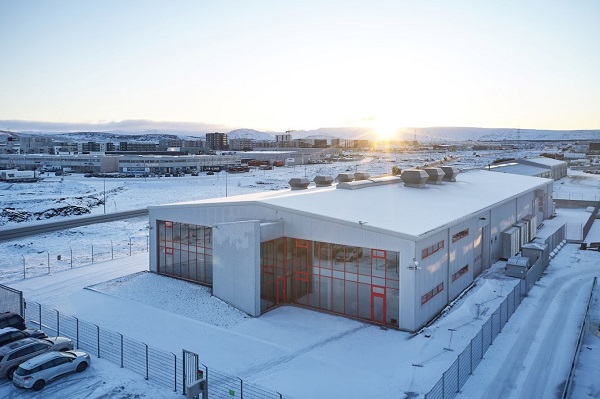
source: atNorth
Fortunately, there are steps that businesses can take to future-proof themselves. Firstly, look to utilise newer data centres that are built from the ground up with these high density, high performance applications in mind. This means that access to data ecosystems can be constructed within days, allowing businesses to get their product to market significantly faster. The data is protected by encrypted links that allow the data centre to act as an extension of the company’s own storage infrastructure.
Additionally, GPU as a service (GPUaaS) technology means the offering is entirely scalable, so that as business requirements change the infrastructure requirements can be increased or decreased as demand fluctuates. This flexible approach is not only more sustainable for businesses whose requirements fluctuate but is more cost effective too.
Another consideration is the environmental cost of HPC. Data centres that are built to cater to these AI workloads require powerful systems with significant cooling requirements that involve the use of large amounts of energy at considerable costs.
Many businesses make pledges around their sustainability or carbon neutral commitments but this is often hard to implement and substantiate. The bulk of net-zero claims are based on buying from renewable energy suppliers that might use certificates like guarantees of origin (GoOs) and power purchase agreements (PPAs). Unfortunately, some suppliers are able to provide these despite neither buying nor generating renewable energy – a type of ‘greenwashing.’ But there are other ways of physically reducing the amount of energy used.
In fact, cooling is responsible for 39 percent of the total electricity cost of most data centres. This means it is equally important that organisations consider the physical environment where their data centre sites are located. Using data centres in countries that have a cool and more consistent climate will ensure temperature and humidity levels within the data centre are maintained more efficiently, while also reducing energy outputs and pollution, and ultimately decreasing the overall carbon footprint and cost to the business. Sweden, for example, benefits from a circular economy whereby any excess energy like the heat generated in data centre facilities can be repurposed via heat recovery systems to heat homes in local communities. This significantly increases energy efficiency and lowers costs for clients.
It seems the future of AI capability is exciting, forward thinking and revolutionary. And yet, as energy costs continue to increase, the financial and environmental impact of these technological advancements are set to skyrocket. To go some way to reduce this impact, businesses must look towards migrating their IT workloads to purpose built data centre sites, especially where the processing of large amounts of compute over high density workloads is needed. Utilising sites that are located in cooler climates and use green and renewable energy will also go a long way to mitigate the ecological impact involved in AI and HPC.
Guy D’Hauwers is global director – HPC & AI, atNorth, a Nordic data center services company based in Iceland.