Jim Collins writes that a research team from Argonne National Laboratory and the University of Chicago is using the Mira supercomputer to investigate the effectiveness of dynamically downscaled climate models.
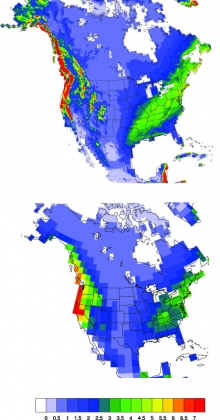
Average winter precipitation rate (mm per day) for a 10-year period (1995 to 2004) as simulated by a regional climate model with 12-km spatial resolution (top) and a global climate model with 250-km spatial resolution (bottom).
Credit:
Jiali Wang, Argonne National Laboratory
From agricultural disruptions in Illinois to rising sea levels on the East Coast, changing climate conditions have far-reaching impacts that require local and regional attention.
Armed with more accurate regional climate projections, policymakers and stakeholders will be better equipped to develop adaptation strategies and mitigation measures that address the potential effects of climate change in their backyards.
While global climate models are used to simulate large-scale patterns suitable for weather forecasting and large-area climate trends, they lack the level of detail needed to model conditions at local and regional scales.
With a method called dynamical downscaling, researchers can use outputs from coarse-resolution global models to drive higher-resolution regional climate models. The enhanced resolution allows regional models to better account for topographic details, while also improving the ability to simulate surface variables such as air temperature, precipitation, and wind.
As part of an ongoing study at the Argonne Leadership Computing Facility (ALCF), a DOE Office of Science User Facility, a research team from Argonne National Laboratory and the University of Chicago is using supercomputing resources to investigate the effectiveness of dynamically downscaled climate models.
The goal of this work is to address a long-standing question in the scientific community regarding the value of downscaling,” said V. Rao Kotamarthi, Argonne climate and atmospheric scientist. “Through our research, we hope to validate a tool that can be used to get climate models to the scales needed for local and regional projections.”
Bringing regional climate into focus
The Earth’s climate is an inherently complex system dependent on multiscale interactions between the atmosphere, oceans, land masses, and the biosphere. Modeling all of these subsystems to generate accurate climate projections in a reasonable amount of time necessitates the use of high-performance computers.
Through a Director’s Discretionary allocation at the ALCF, Kotamarthi’s team is using Mira, a 10-petaflops IBM Blue Gene/Q supercomputer, to simulate several decades of climate conditions over North America at unprecedented spatial and temporal resolution.
The researchers leveraged the open-source Weather Research and Forecasting (WRF) model, a widely used numerical weather and climate prediction tool, to develop a regional climate model with a spatial resolution of 12 kilometers (i.e., the model covers North America with a grid of 12-kilometer boxes).
This is a vast improvement over global models, which typically operate with a spatial resolution of anywhere from 100 kilometers to 300 kilometers. With a total of about 10 million grid cells, the regional model allows the researchers to zoom into specific areas for a more detailed look at regional climate across the continent.
If you’re trying to model the weather or climate over a mountainous region, a global model will not capture the details of its complex terrain,” said Argonne postdoctoral researcher Jiali Wang, a member of the research team. “Our high-resolution regional model can capture those fine details, which is very important because climate systems are greatly affected by regional terrain.”
Temporal resolution, on the other hand, has to do with the size of the model’s time steps, or how often it calculates all of the parameters (temperature, wind speed, etc.). Global models generally run with simulation time steps of about 30 minutes and save the output every 24 hours of simulated time. The Argonne team’s regional model runs with simulated time steps of 40 seconds and saves the output every three simulated hours—a timescale that gives them the ability to explore climate changes that occur on a diurnal scale, such as thunderstorms or urban heat islands.
With Mira, approximately 1 million core-hours are needed to run a one-year simulation on 512 nodes (with each node having 16 cores). In this configuration, a 100-year simulation can be carried out in approximately 50 days.
If you asked me five years ago to run a high-resolution regional climate model for 100 years of simulations over such a large domain, I don’t think it would have been feasible,” Kotamarthi said.
Comparing simulation results to real-world data
The evaluation of regional climate models against observational data is an important step in building confidence in their ability to predict future climate conditions. In a paper published in the Journal of Geophysical Research – Atmospheres, Kotamarthi’s team assessed their regional model’s performance for one specific variable—precipitation—to see how well it captured spatiotemporal relationships.
Climate systems are space-time structures that move and change with time, so it’s critical that models simulate this behavior as accurately as possible,” Kotamarthi said.
Their study involved calculating two measures for precipitation patterns over North America: (1) spatial correlation for a range of distances and (2) spatiotemporal correlation over a wide range of distances, directions, and time lags.
They found that the correlations in the regional model output show similar patterns to observational data, and exhibit much better agreement with the observations than the global model in capturing small-scale spatial variations of precipitation, especially over mountainous regions and coastal areas.
In addition, the researchers did more of an apples-to-apples comparison by “regridding” the data from their 12-kilometer regional model to match global models with spatial resolutions of 100 kilometers and 250 kilometers. These comparison also showed that regional model retained more information and matched observational data more closely than the global models did.
Our research shows that downscaling does add significant value to the simulations at a regional scale,” Kotamarthi said. “The results provides justification for the use of downscaled regional models to represent the statistical characteristics of precipitation accurately.”
Getting up to speed on Mira
When the team transitioned their work from Intrepid (the ALCF’s previous generation supercomputer) to Mira in 2013, they experienced a slowdown in I/O performance.
To provide an immediate solution to the problem, Ray Loy, ALCF application performance engineer, created a job script that allowed the team to perform ensembles (a group of simulations bundled together to run as one larger job). This approach enabled each simulation to run at a scale where I/O did not hinder performance.
We are now able to submit several simulations at one time, which allows us run simulations two to four times faster than before,” Wang said.
At the same time, ALCF performance engineering staff helped Kotamarthi’s team by developing a benchmark to determine the root cause of the problem. After discovering redundancies in how their code was reading the boundary data, they developed new parallel I/O algorithms that demonstrated the ability to speed the reading of this data by a factor of 10 in benchmark tests.
We are now working with the WRF (Weather Research and Forecasting) code developers to see how these new algorithms can be incorporated into the model, so all researchers using WRF can benefit from the improvements,” said ALCF computer scientist Venkat Vishwanath.
With the job script solution in place, the researchers were able to complete simulations of several decades, including two historical time periods for their spatiotemporal correlation study, as well as future time periods to predict how precipitation patterns will likely change in coming decades.
They are planning to make this data publicly available, so local and regional decision makers can use the results to inform climate change adaptation strategies. The results will also be useful the scientific community as researchers are already planning to use the data to further study the impact of changing climate conditions on everything from extreme weather to power generation.
As a next step, Kotamarthi and his team are increasing the size of the ensembles with slightly different initial conditions, so they can explore internal variabilities in their model. They also plan to incorporate outputs from additional global climate models to enable the regional model to simulate a larger range of possible outcomes, which will enhance their understanding of uncertainties in future climate projections at the regional scale.
This work is supported under a military interdepartmental purchase request from the U.S. Department of Defense’s Strategic Environmental Research and Development Program (SERDP), RC-2242, through U. S. Department of Energy contract DC-AC02-06CH11357. Computing time at the ALCF is supported by the DOE Office of Science.
Source: Argonne