This sponsored post from Lenovo explores innovation at the convergence of HPC and AI, including early detection of prostate cancer, mitigating the impact of deforestation, preventing visual impairment, and more.
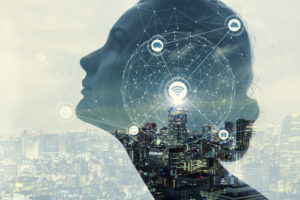
The reality is, AI requires commitment- from investment to the core of your organizational strategy. (Photo: Shutterstock/By metamorworks)
By the end of 2019, worldwide AI spend is expected to reach $35 billion and more than double by 2022, according to IDC. While AI market projections may be speculative, there’s a general consensus the investment will be significant, and the impact will be transformative. There are skeptics that believe the marketing hype will not match reality, and this notation is substantiated by the Gartner Hypecycle, which has AI at the peak of inflated expectations. Will AI truly be as transformative as many envision, or will implementation simply be a lofty but unattainable goal for most companies? In all likelihood, it will be in between those two extremes. As an industry we’ll have to reset expectations as to how enterprises will truly utilize AI and the real value it will enable.
Separating Hype from Reality
The truth is, AI is not easy. There is a diverse set of skills and technical requirements from data collection, data cleansing, model development, and implementationand operationalization. The reality is, AI requires commitment — from investment to the core of your organizational strategy. You may be thinking, if AI is difficult, then why do we hear of new research and discovery nearly every day powered by AI? Moving from proof-of-concept to deploying in a real-world environment can create significant challenges from infrastructure and talent to regulatory restrictions. This process of operationalizing AI is one of the greatest challenges technical professionals face, according to Gartner. This includes building data pipelines, creating ETL scripts, defining inference architecture and model deployment in a DevOps environment, just to name a few.
Operationalizing AI may look vastly different from one organization and use-case to another.
AI Applied to Research
That is not to diminish the amazing research that fuels the AI movement and inspires us to use our imagination on how we apply this technology. There are a number of ground-breaking research initiatives that are only possible by leveraging AI along with HPC systems. As we move toward the exascale era, supercomputing will unlock new research and discovery opportunities for AI, as well as traditional HPC workloads. AI is also driving additional grant funding, interest from a broader researcher community and even private sector investments.
Here are just a few AI research projects where Lenovo is deeply involved:
Early Detection of Prostate Cancer
The University of Chicago is collaborating with Lenovo to leverage AI technology to improve the early detection of prostate cancer. Prostate cancer is one of the most common forms of cancer effecting men, and early detection can significantly improve patient outcomes. By utilizing AI technology to analyze multiparametric MRI images, we can transform the patient screening process — minimizing unnecessary prostate biopsies and surgeries.
Mitigating the Impact of Deforestation
byteLAKE is working on an initiative that leverages computer vision to analyze images captured by drones, tracking the survival rate of newly planted trees. This initiative is empowering reforestation efforts that will ultimately impact global climate change in a positive way.
Preventing Visual Impairment
Through a collaboration with Lenovo, the Barcelona Supercomputing Center (BSC) has set out to explore how AI can improve the accuracy of the retina screening process by nearly 10 % and potentially detect a retinal disease sooner. AI technology further increases the likelihood of early detection by allowing patients in underserved populations to self-administer an initial screening in a matter of minutes, using a smartphone.
Curbing the impact of Climate Change
North Carolina State University’s (NCSU) research program, in partnership with Lenovo, is actively invested in addressing the effects of climate change on the agricultural ecosystem. To help minimize the disruption to food production, it’s critical that farmers are able to prepare for anticipated regions that will experience drought (or flooding) which will negatively impact crop growth. Led by Dr. Ranga Raju Vatsavai, associate professor in the computer science department and associate director of Center for Geospatial Analytics, NCSU’s research team is leveraging innovative Geospatial Image Analysis technology to map and monitor croplands and preemptively identify local areas that will be affected by flooding or droughts.
A quick search online will give you many other examples of AI being used to tackle some of humanity’s greatest challenges, but these examples can leave enterprises and other non-research organizations wondering: What does AI mean for me?
Enterprise AI Adoption
Even if you are not tackling disease detection or climate change and you don’t have the means to invest in an exascale system or massive HPC cluster, enterprises can still benefit from the high-end technology being pioneered for HPC and the research advances in artificial intelligence. As we have seen in the past, HPC and hyperscale have paved the way for much of the technology making AI accessible today, from parallel processing, high-speed interconnects, accelerators and open-source software, to containerization and APIs. We believe certain exascale technology will become pervasive, making its way into enterprise and empowering new capabilities. We define this concept as exascale to everyscale. Beyond HPC paving the way for enterprises on the technology front, we’ll also see AI use-cases such as computer vision techniques being leveraged by enterprises. For example, our AI Research lab developed a model to detect liver tumors, and we are now able to provide that as a pre-trained model in the latest version of our LiCO (Lenovo intelligent Computing Orchestration) AI Platform. From knowledge sharing through academic research publications, new AI architectures and startups spun up from research initiatives, enterprises have a lot to gain from the work being pioneered by HPC.
Key ingredients for success
According to O’Reilly Media and a recent MIT survey, nearly 80% of organizations have not moved their AI projects past the PoC stage, meaning most organizations are still in the experimentation phase or have not launched their AI initiative. For organizations interested in AI, there’s much to consider. Whether you haven’t started your AI journey or you are already working on implementing your projects, here are a few things to think about to ensure success.
Executive buy-in — Although this is probably common sense, it is important for the leadership of the organization to see the value in the AI initiatives.
AI Data Readiness — What data do we have, and is it ready for a data scientist to use? These are the first questions all organizations considering AI implementation should ask themselves. Data cleansing/wrangling is typically the most labor-intensive part of the data science process, so it is always better to start with data that has the highest readiness score to ensure time spent on data cleansing is minimized. Enterprises also need to think through the infrastructure to warehouse and transport their data, and their ETL process. This is typically an iterative process, since you may realize further refinement of your data is needed once a data scientist begins to build the initial model. A good place to start is with descriptive analytics before moving to AI. Ask yourself where you are utilizing data analytics within your organization to drive decision making.
Access to Talent — Data scientists are in high demand with most job sites reporting around a 30% year-over-year increase in demand, one of the lowest unemployment rates, and a median salary nearly double the national average in the U.S. Attracting top talent in AI can be a significant challenge for enterprises. Before hiring a team of data scientists, organizations should consider developing the AI strategy and run an initial PoC to understand the level of investment they will need to make. Technology providers can help with this process.
Implement & Operationalize — Consider the pipeline for deploying and updating models, the data management strategy, existing DevOps environment and more importantly, how to incorporate AI into the organization’s decision making process (the value the organization places on data driven decisions). This may require a culture shift.
Start Simple and Scale — Start with a less complex business case that has a straightforward ROI. Also, consider what skills exist internally and where there are gaps. Enterprises can also leverage existing infrastructure to get started and then invest in specialty infrastructure once the business case has been proven. Also, the use-cases that cause the least disruption to current business processes are more likely to be successful.
What’s next for AI?
As we see more projects move into operationalization and an increase of inference workloads, organizations will need to think through how their AI strategy will converge with IoT and edge deployment. The impact of edge and IoT will be driven by the specific use-cases and may not be as significant for all industries. Also, the adoption of 5G technology and new architectures such as federated learning may change the mix of core/cloud/edge, but most use-cases will inevitably utilize a hybrid architecture that will span a diverse set of technologies.
To address the growing demand in emerging technologies, we have recently announced that we will be launching our largest portfolio in telecom and edge/IoT and have recently also announced our Lenovo ThinkSystem SE350 edge server that supports an NVIDIA® Tesla® T4 in a compact, ruggedized platform. We have also recently announced our support of the NVIDIA EGX, an accelerated computing platform that enables companies to harness AI at the edge — to perceive, understand and act in real time on massive data flows from billions of sensors in store aisles, on factory floors, and beyond.
We see the value in providing a strong partner ecosystem to support the diverse needs of our customers and are investing in the technologies and partnerships that are needed to ensure that our customers’ AI initiatives are a successful and worthwhile endeavor for their organization.
This sponsored post is brought to you by Lenovo.