The is the third entry in a five-part insideHPC series that takes an in-depth look at how machine learning, deep learning and AI are being used in the energy industry. Read on to learn how edge computing is playing a role in operating drilling rigs, ensuring pipeline integrity and more.
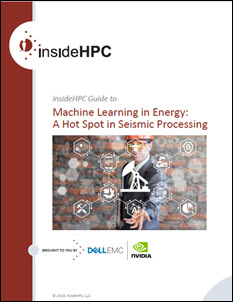
Download the full report.
Data at the Edge
As part of the overall exploration workflow that then leads into production, a number of areas exist in which computing at the edge greatly benefits the process and can lead to reduced time to make decisions. For example, if the terabytes of data generated at the source can be filtered locally, less data must be sent over unreliable or non-existent networks that may exist in areas far away from reliable communication devices. The advantage is not just in filtering data at the edge of the network, but also in relying on previous patterns and known data sets that are available. Sophisticated ML algorithms can be trained to recognize when data is reliable or when it could be discarded without human interaction.
ExxonMobil is using AI robots for ocean exploration to look for natural seeps of oil, which can contribute to protecting the environment as well as serve as indicators for new energy resources.
Drilling rigs are very complex to build and operate. Once built, thousands of sensors must be monitored and corrective actions must be taken depending on readings. Through edge computing, deep learning and modern interfaces (voice controls), a more accurate understanding of the operation of the drilling platform can be obtained, and problems can be identified and fixed before a major issue surfaces.
Pipeline integrity is also an area where experiences from previous investigations are used. With miles of pipelines contained within and external to a drilling rig, an early understanding of possible failure modes can result in significant cost and environmental savings later. Using a combination of imaging from cameras and experiences gathered in the past, systems that employ edge computing and ML alert operators to possible upcoming failures. Over time, corrosion of the pipelines can cause a major environmental disaster. However, by using knowledge from material scientists, engineering and computational fluid dynamics domains, corrosion can be more reliably detected and corrective action performed.

With miles of pipelines contained within and external to a drilling rig, an early understanding of possible failure modes can result in significant cost and environmental savings later.
In addition to cameras that record the construction of a complex system, robots and drones record the conditions in areas where humans cannot go. These additional points of view in both space and time lead to a better understanding of the physical system that must remain intact and productive under harsh conditions for many years to come. Comparing an existing image to a previous image for each pixel in the image can lead to new insights. The subtle changes that deep learning algorithms find are an added tool to extend and secure the life of these systems and can reduce the chance of a crack or leak causing significant stoppages to the production system, or worse.
Over the next few weeks, this insideHPC Guide series will also cover the following topics:
- Opportunities Abound: HPC and Machine Learning for Energy Exploration
- Energy Companies Embrace Deep Learning for Inspections, Exploration & More
- Computing Environments Options for Energy Exploration and Monitoring
- Determining Where & How to Adopt Machine Learning Technology
Download the full report, “Machine Learning in Energy: A Hot Spot in Seismic Processing,” courtesy of Dell EMC and Nvidia, to learn more about how machine learning is being used to move the energy industry forward.