By Joy King, VP, Vertica Product Management & Product Marketing
Remember when Hadoop was going to replace the enterprise data warehouse (EDW)? That seemed to be where things were heading. The EDW was a closed architecture, an appliance, hard to integrate, plus the EDW was struggling to keep up with all the innovations coming out of the open source community. Designed for structured data, the EDW couldn’t handle all the unstructured or semi-structured data that was becoming important for enterprises to manage. And on top of all this, the rising cost of appliance-based EDWs was getting hard to justify. By contrast, Hadoop was free, fully scalable, and open source – and a sexy addition to any resume!
It didn’t take long for reality to take the shine off Hadoop’s halo. Many queries failed, and performance was a big sacrifice because while the data lake was designed for highly distributed storage, it wasn’t optimized for the consistent performance and concurrency required for big data analytics. Despite multiple concentric projects like Impala, Yarn, Hive, and many others, the challenges continued but so did the foundational value of low cost distributed storage.
Since the launch of Hadoop 14 years ago, the cloud has introduced innovative storage options for the data lake, offering the same boundless capacity that Hadoop on commodity hardware provided. The data lake concept is still alive because the need for data storage is only expanding, whether that’s using cloud-based storage or traditional Hadoop distributions. On the EDW side of this story, the need to corral massive data sets (but still direct them to a single data repository) and apply modern AI techniques in the cloud, or in hybrid environments, has proven the need for data governance, security, and reliable performance despite the fact that the former appliance model has become obsolete.
So where does the big data industry stand today, given the great data lake / EDW divide?
Put data where you like, but unify the analytics
We believe that the data lake and the EDW can – and must – work side by side. It doesn’t matter whether data is stored in a lake, a cloud, on-premises, or any combination of those. What matters is that we’re able to unify the analytics – which is what makes all this data worth storing in the first place. We call this concept the “Unified Analytics Warehouse.”
Data warehouses bring a lot of strengths to the UAW – security, resiliency, performance, and data governance. At the same time, data lakes offer very efficient, distributed file storage and provide for semi- and unstructured data. With access to very large data sets, machine learning finally becomes operational. Now that we can wrap our massively parallel analytical processing around petabyte-scale data sets, we’re discovering patterns and trends we can trust, that are real and actionable.
And to do that, you need to span data locations with a unified approach to the analytics.
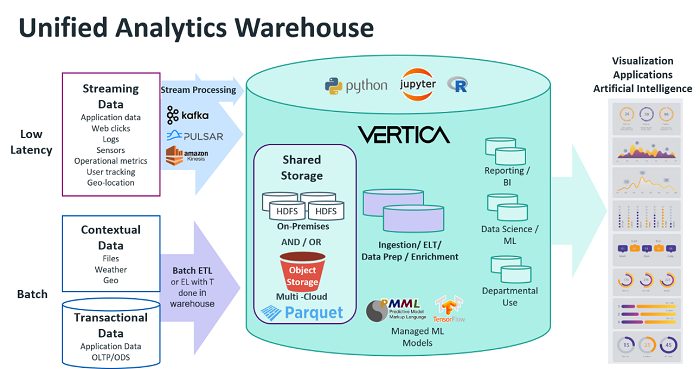
The Vertica Unified Analytics Warehouse spans multiple data types and storage.
Analytics for multiple communities
The focus on unified analytics elevates the question from “which technology do we use?” to “which community do we serve?” Before, we had the data warehousing and the SQL community, or we had the data lakes and the R, Python, Jupyter notebooks community. Now we can serve both communities effectively without forcing one to move to a new set of tools.
We need to stop our fixation with “data in one place.” The days of the single data repository are behind us. We can’t expect our data to be in one cloud, one format, one restricted location, especially in a world where “data egress” fees and intentionally slow data export networks are a reality. If you try doing that, you’ll incur so much data management time and cost that you’ll squander the savings even before you even get to the analysis stage.
To put it simply, the goal is to unify, analyze, and act, because predictive analytics and proactive action is the definition of business success.