By Adrian Cockcroft, Partner & Analyst, OrionX
This post is an update to the predictions story I wrote after attending SC22, which was first published as a story on insideHPC. In that post I talked about the initial emergence of CXL as an architectural trend. At SC23, there was a lot more activity and support for CXL, a general sense that it has become the “only game in town,” but that its more advanced features are going to take longer to develop than we hoped a year ago.
A Cloudy Future for Top500?
Before looking at CXL, here’s my take on the updates to the Top500 list of the world’s fastest supercomputers. Last year, the first exascale result was posted by the HPE/Cray “Frontier” AMD-based system at 1.1 exaflops (now it’s at 1.2 exaflops). At the time, the even bigger Intel-based “Aurora” system, using the HPE-Cray Shasta system architecture, was running late, and this year Aurora’s partial installation run was a new entrant in second place on the list at 585 petaflops. Aurora will undergo several more months of bring-up work, but expect it to exceed Frontier’s number when fully commissioned.
One comment I heard at the conference is that while the High Performance LINPAC (HPL) benchmark isn’t that useful as a real-world workload, it is a useful stress test that requires the system to be well sorted out before you can get a good result.
The third place result was a run by Microsoft Azure on its “Eagle” system, with Intel CPUs, NVIDIA H100 GPUs and Infiniband interconnect, at 561 petaflops. It’s mostly intended for use as an AI training configuration, but an interesting aspect is that the Azure team said they spent only a few days configuring and running the benchmark. They also said performance was still scaling well when they stopped allocating hardware for the final result. This is a huge contrast with the time taken to get “traditional” supercomputers working. While it’s true that Eagle’s software stack and storage makes for a different, less time-consuming, setup, I think this highlights the difference between “hand-crafted” data center supercomputers and cloud deployments. I expect to see the Top500 list overrun with cloud-based entrants in future years.
The Crossover Between AI and HPC
The investment in dedicated HPC supercomputers has been eclipsed by the investment in AI training supercomputers over the last few years. The hardware is similar enough that AI training has become part of the supercomputer workload mix. However the latest generation NVIDIA GPU architectures are optimized for AI, with low precision floating point and dedicated “AI transformer” accelerators. They are still good at running traditional HPC codes, but a purpose-built, HPC-oriented architecture would optimize the silicon layout differently.
As I said last year, I still think we’ll start to see more custom, HPC-optimized CPU and accelerator architectures in future years, but they haven’t turned up yet. Meanwhile, GigaIO has figured out how to add more GPUs on a single node (most vendors stop at 8 GPUs per node), recently doubling from their 32 GPU SuperNode to a 64 GPU SuperDuperNode, which I thought was a fun naming scheme. Maybe we’ll end up at the “SuperDuperComputing Conference” in a few years.
Chiplet Standardization
Last year the UCIe chiplet standard had just been launched, and since then just about everyone has joined it, about 130 companies. The idea is that to build a complete CPU or GPU you don’t have to put it all on the same chip from the same vendor. Instead, you can use the UCIe standard to innovate in your own chiplet, then surround it with chiplets for memory and IO from a range of vendors.
They are all mounted on a substrate that provides much better performance than interconnecting via a PCB. Chiplets aren’t new, they are already used in the latest designs, but aligning everyone on the same substrate interface standards will allow more innovation. Companies like Intel will assemble chiplets from multiple vendors onto substrates to form the finished device. This is also part of Intel’s move to compete more directly with TSMC and build custom chips rather than focus on their own designs.
Last year I made the prediction that custom CPU/GPU/Vector designs optimized for HPC workloads would emerge, and UCIe makes that a lower cost proposition to implement. ARM-based CPUs from SiPearl and NVIDIA are a step in this direction, but I still think Fugaku is more likely to be the prototype of whatever dedicated HPC architecture comes next, and that it will be implemented using UCIe chiplets.
Speeds and Feeds
CXL runs on the physical substrate that’s been developed by the PCI standard, and one big update I saw since last year is that the PCI7.0 spec is under development, with another doubling in speed by optimizing the PCI6.0 hardware spec to run at twice the frequency. Each PCI7.0 connector will carry 256GBytes/sec, with separate connectors in each direction to make up a channel.
The currently available Intel Sapphire Rapids 4th Generation Xeon CPU supports four PCI5.0/CXL1.1 16-lane channels at a quarter of that data rate. Intel 5th generation Emerald Rapids and Granite Rapids CPUs will support PCI5.0/CXL 2.0. The NVIDIA Grace CPU was stated to support CXL2.0 when it was announced, but it isn’t mentioned in the latest NVIDIA Grace documentation. CXL is only mentioned as a supported protocol in the NVIDIA chip-to-chip (C2C) interconnect.
There were demonstrations of PCI6.0 on the SC23 show floor and plans for next-generation CPUs call for four PCI6.0/CXL2.0 channels. That’s a terabyte per second of I/O bandwidth that can be repurposed via CXL as memory bandwidth, on top of whatever memory interface they have. It seems plausible that an end game is to have a relatively small amount of dedicated HBM to provide the highest performance CPU and GPU memory, with CXL for capacity memory that can be re-allocated and shared where it is needed, using conventional DRAM. This is another twist on the old non-uniform memory access (NUMA) model that has been around for many years and has good operating system support.
Allocating and Sharing CXL Memory
The CXL consortium announced a new CXL3.1 version of the specification at SC23. It extends the features of the fabric management aspects and adds some security and trust capabilities that will be needed in multi-tenant deployments. I did not have time to visit all the booths in the SC23 expo that had something to do with CXL, but I did see good progress overall. It also was clear that the more advanced fabric management features are a bit further out than we were hearing last year. We’ll see PCI6.0/CXL3.0 with multi-level switching first, then it seems likely that real deployments of fabric management will skip CXL3.0 and be based on the new CXL3.1 spec in 2–3 years, as we need time for new CPU designs to ship with CXL3.1.
The general idea is that there can be a large amount of CXL memory in a rack that is shared across several nodes as needed. The initial CXL1.1-based systems don’t support switching, but CXL2.0 switches are available that support pooled memory devices. The memory would need to be allocated to a single host when it boots. CXL3.1 will allow multiple levels of switches and a dynamic fabric that could allocate the same memory to multiple hosts as a shared resource, change the fabric layout and add nodes and change memory allocation while the systems are running. Many things need to come together to make that work, but it increasingly appears to be the end point that many people are working towards.
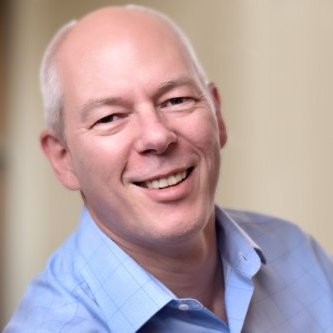
Adrian Cockcroft
Takeaway
I’m happy with progress against the predictions I made last year, I had a good time at SC23, met with old friends and made some more new ones. I also took a good look at the research poster area and spent some time looking for information on actual communication workload characteristics that will be a subject of another post.
Adrian Cockcroft is a Partner & Analyst the OrionX technology consulting firm.